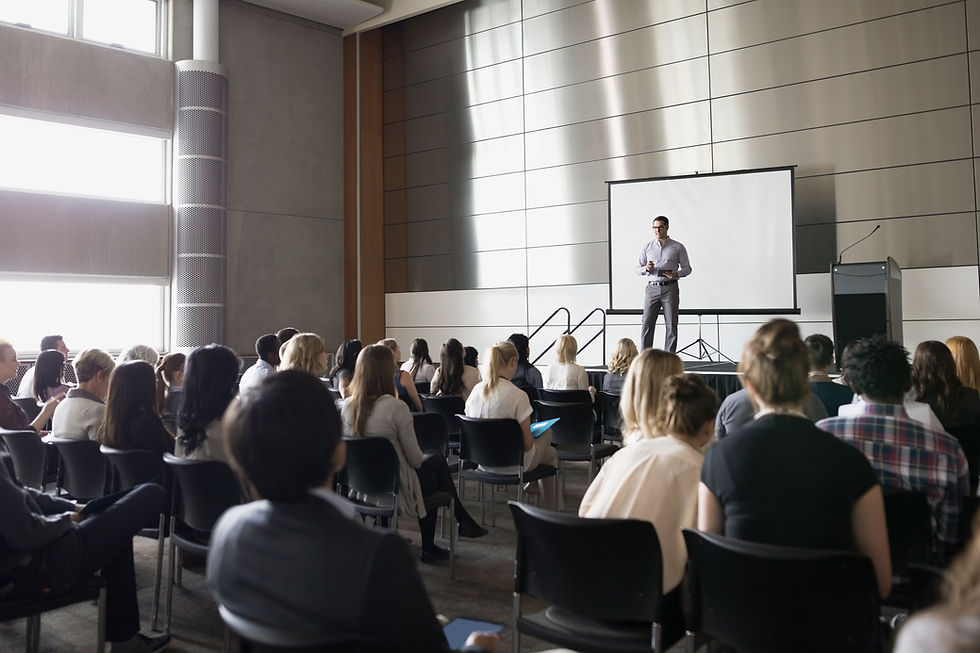
WCCI 2022 Special Session on
Transfer Learning under Weak Supervision
This special session aims to provide a forum for researchers in the transfer learning field to share the latest advantages in theories, algorithms, and applications on deep transfer learning under weak supervision. Specifically, this session will focus on deep transfer learning with inaccessible domains, deep transfer learning with incomplete domains, deep transfer learning with inhomogeneous domains, and deep transfer learning with inaccurate domains. These research directions include several topics in the field of transfer learning, such as source-data-free domain adaptation, open-set domain adaptation, heterogeneous domain adaptation, wildly domain adaptation, domain generalization, few-shot domain adaptation, and universal domain adaptation.
Background
With the development of deep neural networks, researchers can extract good data representations using well-trained deep neural networks (e.g., pre-trained ResNets) and use the knowledge contained in these representations to help address problems in many downstream tasks. This knowledge transferring process is also known as deep transfer learning. In deep transfer learning, we have source and target domains and aim to use knowledge in the source domains to address problems in the target domains.
Traditional deep transfer learning methods often assume that the above knowledge transferring process is guided by strong/perfect supervision. For example, in unsupervised domain adaptation, we assume to be able to access perfect-labeled data in the source domains and plenty of data in the target domain. However, this assumption does not hold in many realistic scenarios: e.g., we might not have the right to access data in the source domains (due to privacy concerns) or we might not have considerable budgets to hire experts to get the perfect-labeled data. This motivates researchers to focus on deep transfer learning under weak supervision. For example, deep transfer learning under noisy labels, deep transfer learning under data-privacy protection, and deep transfer learning under few data observations.
Aims
The main goal of this special session is to provide a forum for researchers in the transfer learning field to share the latest advantages in theories, algorithms, and applications on deep transfer learning under weak supervision. Specifically, this session will focus on deep transfer learning with inaccessible domains, deep transfer learning with incomplete domains, deep transfer learning with inhomogeneous domains, and deep transfer learning with inaccurate domains. These research directions include several hot topics in the field of transfer learning, such as source-data-free domain adaptation, open-set domain adaptation, heterogeneous domain adaptation, wildly domain adaptation, domain generalization, few-shot domain adaptation, and universal domain adaptation.
Detailed Descriptions
Due to the considerable cost of labeling data and the increasing requirements of protecting data privacy, it is unrealistic to assume that we can always use perfect labeled data in source domains or have enough data in the target domains during transfer learning. In the real world, we have to use imperfect data in source domains (e.g., data with label noise) or use only models trained with source data to perform the transfer learning across the source and target domains. Thus, it is good timing to provide a forum for researchers in the field to share the latest advantages in theories, algorithms, and applications on transfer learning under weak supervision.
This special session focuses on four types of imperfect scenarios that traditional transfer learning methods face: inaccessible source/target domains, incomplete source/target domains, inhomogeneous source/target domains, and inaccurate source domains. In the following, the above imperfect scenarios are introduced in detail.
1) Inaccessible source/target domains. Due to the data-privacy concerns, data owners do not allow us to access their data in this scenario. The related research topics include but are not limited to:
Hypothesis Transfer Learning (inaccessible source domains)
Source-data-free Unsupervised Domain Adaptation (inaccessible source domains)
Transfer from A Zoo of Models (inaccessible source domains)
Few-shot Hypothesis Adaptation (inaccessible source domains)
Domain Generalization/Out-of-distribution Generalization (inaccessible target domains)
2) Incomplete source/target domains. In this scenario, the information in the source/target domains might not be completed due to the domain-index missing or domain-label misaligned, or domain-data scarcity. The related research topics include but are not limited to:
Source/target Component Domain Adaptation (domain-index missing)
Open-set Domain Adaptation (domain-label misaligned)
Partial Domain Adaptation (domain-label misaligned)
Universal Domain Adaptation (domain-label misaligned)
Few-shot Domain Adaptation (domain-data scarcity)
3) Inhomogeneous source/target domains. In this scenario, domains’ feature spaces are not homogeneous. For example, the source domains only contain images regarding animals while the target domains are descriptions (text) regarding these animals. The related research topics include but are not limited to:
Heterogeneous Unsupervised Domain Adaptation
Heterogeneous Semi-supervised Domain Adaptation
Cross-modality Domain Adaptation
Few-shot Heterogeneous Domain Adaptation
4) Inaccurate source domains. In this scenario, data owners do not have perfect labels for their data due to the considerable costs to annotate large-scale datasets. To avoid the cost to hire domain experts to annotate data, data owners will consider using cross sourcing to get data with label noise or hiring low-cost annotators to get data with partial/complementary labels. The related research topics include but are not limited to:
Wildly Domain Adaptation
Weakly Supervised Domain Adaptation
Complementary-label Domain Adaptation
Partial-label Domain Adaptation
Except for the above research topics, we also welcome theoretical papers regarding deep transfer learning or deep transfer learning under weak supervision. The related research topics include but are not limited to:
Theory regarding Unsupervised/Semi-supervised Domain Adaptation
Theory regarding Domain Generalization
Theory regarding Hypothesis Transfer Learning
Theory regarding Universal Domain Adaptation
Theory regarding Heterogeneous/Few-shot/Wildly Domain Adaptation
Theory regarding Integral Probability Metrics
IEEE WCCI 2022 will be held in Padua, Italy, one of the most charming and dynamic towns in Italy. It is located in the Veneto region, in Northern Italy. The historical center boasts a wealth of medieval, renaissance and modern architecture. Here a fascinating mix of historic and new, of centuries-old traditions and metropolitan rhythms creates a unique atmosphere. The medieval palaces, churches and cobbled streets emanate a sense of history. Padua features rich historical and cultural attractions, such as “Prato della Valle”, the largest square in Europe; the famous Scrovegni Chapel painted by Giotto; the Botanical Garden that is a UNESCO Word Heritage; the University of Padua, that is the second oldest university in Italy (1222) celebrating, in 2022, 800 years of history.
Submission Details
It is our great pleasure to invite you to the bi-annual IEEE World Congress on Computational Intelligence (IEEE WCCI), which is the largest technical event in the field of computational intelligence. The IEEE WCCI 2022 will host three conferences: The 2022 International Joint Conference on Neural Networks (IJCNN 2022 – co-sponsored by International Neural Network Society – INNS), the 2022 IEEE International Conference on Fuzzy Systems (FUZZ-IEEE 2022), and the 2022 IEEE Congress on Evolutionary Computation (IEEE CEC 2022) under one roof. It encourages cross-fertilization of ideas among the three big areas and provides a forum for intellectuals from all over the world to discuss and present their research findings on computational intelligence.
Double-Blind Reviewing
The review process for WCCI 2022 will be double-blind, i.e. reviewers will not know the authors' identity (and vice versa). Authors should ensure their anonymity in the submitted papers. In brief:
authors' names should not be included in the submitted pdf;
please refer to your prior work in the third person wherever possible;
a reviewer may be able to deduce the authors' identities by using external resources, such as technical reports published on the web. The availability of information on the web that may allow reviewers to infer the authors' identities does not constitute a breach of the double-blind submission policy.
Note that anonymizing your paper is mandatory, and papers that explicitly or implicitly reveal the authors' identities may be rejected.
Paper Submission
Please read the following paper submission guidelines before submitting your papers:
Each paper should not reveal author's identities (double-blind review process).
Papers will be checked for plagiarism. The Program Committee reserves the right to desk-reject a paper if it contains elements that are suspected to be plagiarized.
Submissions should be original and not currently under review at another venue.
Each paper is limited to 8 pages, including figures, tables, and references. A maximum of two extra pages per paper is allowed (i.e, up to 10 pages), at an additional charge of 100 € per extra page.
All papers must be submitted through the IEEE WCCI 2022 online submission system. For special session papers, please select the respective special session title under the list of research topics in the submission system.
In order for your papers to be included in the congress program and in the proceedings, final accepted papers must be submitted, and the corresponding registration fees must be paid by May 23, 2022 (11:59 PM Anywhere on Earth).
IEEE WCCI 2022 will present the Best Overall Paper Awards and the Best Student Paper Awards to recognize outstanding papers published in each of the three conference proceedings (IJCNN 2022, FUZZ-IEEE 2022, IEEE CEC 2022). The awards will be judged by an Awards Committee and the recipient of each award will be given a certificate of the award and a cash prize.
It is the policy of WCCI 2022 that new authors cannot be added at the time of submitting final camera ready papers. Please ensure that you are following this guideline to avoid any issues with publication.
When you submit papers to our special session, please note that the ID of our special session is IJCNN-SS-18.
If you encounter any problems with the submission of your papers, please contact the conference submission chair.
Paper submission system
WCCI 2022 adopts Microsoft CMT as submission system, available at the following link: https://cmt3.research.microsoft.com/IEEEWCCI2022/
You can find detailed instructions on how to submit your paper here.
LaTeX and Word Templates
To help ensure correct formatting, please use the IEEE style files for conference proceedings as a template for your submission. These include LaTeX and Word style files.
Violations of any paper specification may result in rejection of your paper.
Manuscript Style Information
Only papers in PDF format will be accepted.
Paper Size: A4 (210mm x 297mm).
Paper Length: Each paper should have 6 to MAXIMUM 8 pages, including figures, tables and references. A maximum of two extra pages per paper is allowed (i. e., up to 10 pages), at an additional charge of 100 € per extra page.
Paper Formatting: double column, single spaced, #10 point Times Roman font. Please make sure to use the official IEEE style files provided above.
No page numbers please. We will insert the page numbers for you.
Note: Violations of any of the above specifications may result in rejection of your paper.
Important Dates
Paper submission: January 31, 2022 (11:59 PM AoE) STRICT DEADLINE
Notification of acceptance: April 26, 2022
Final paper submission: May 23, 2022
Conference: 18 - 23 July, 2022

ORGANISERS
Get in Touch
If you have questions about the submission/registration process, don’t hesitate to reach out using the following email.
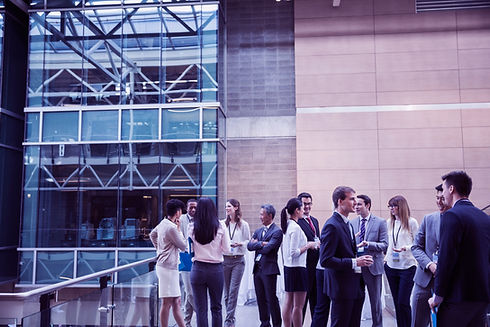